Data Analysis with R Training
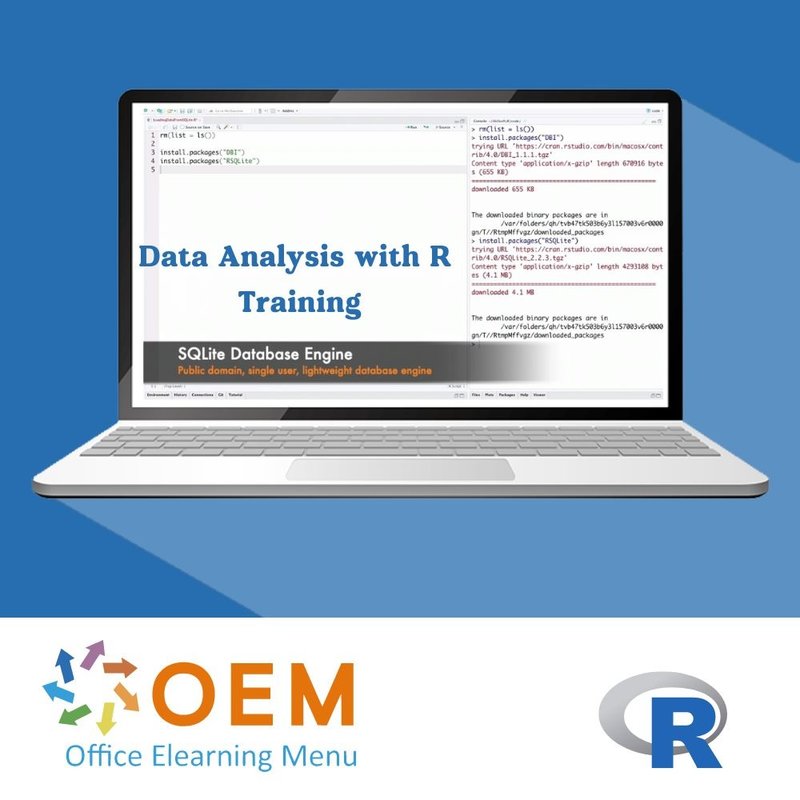
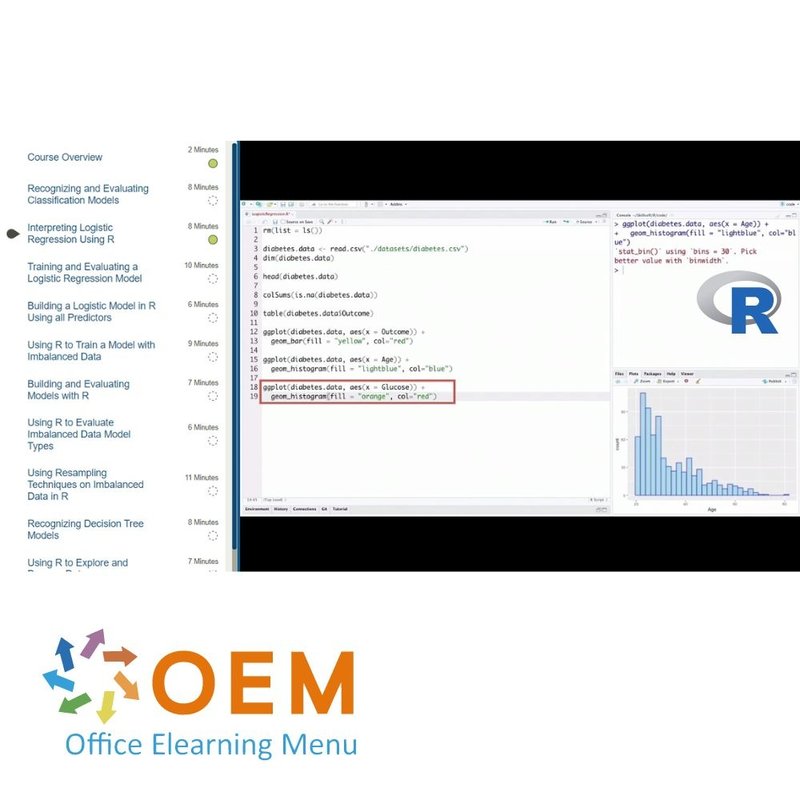
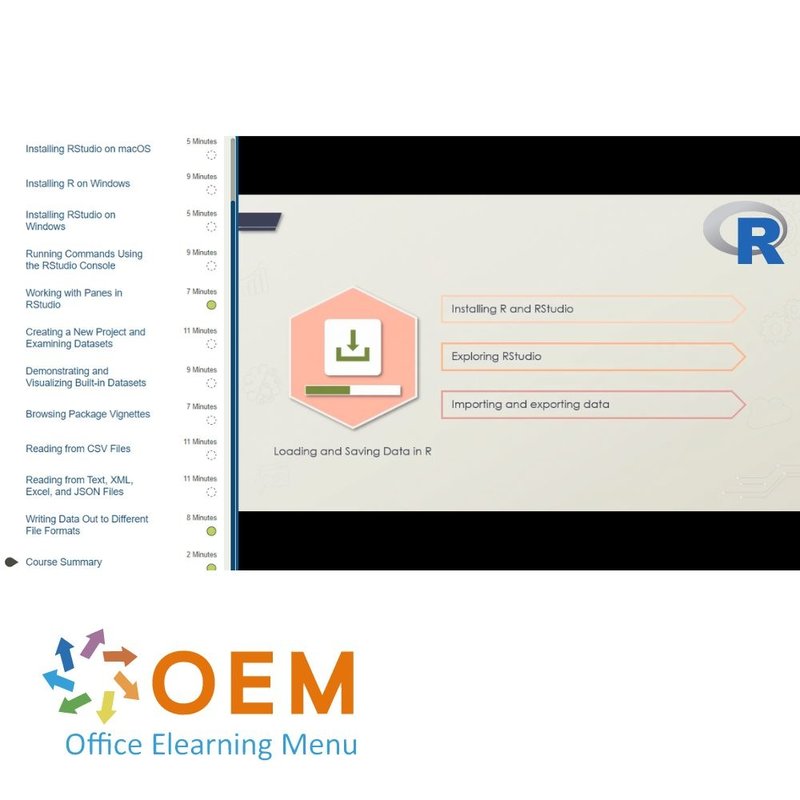
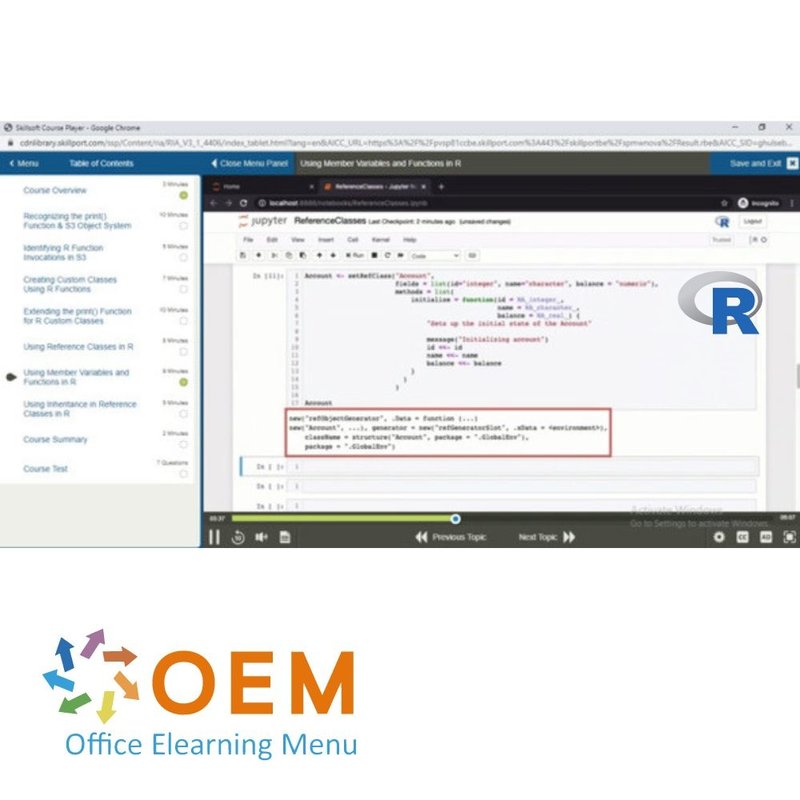
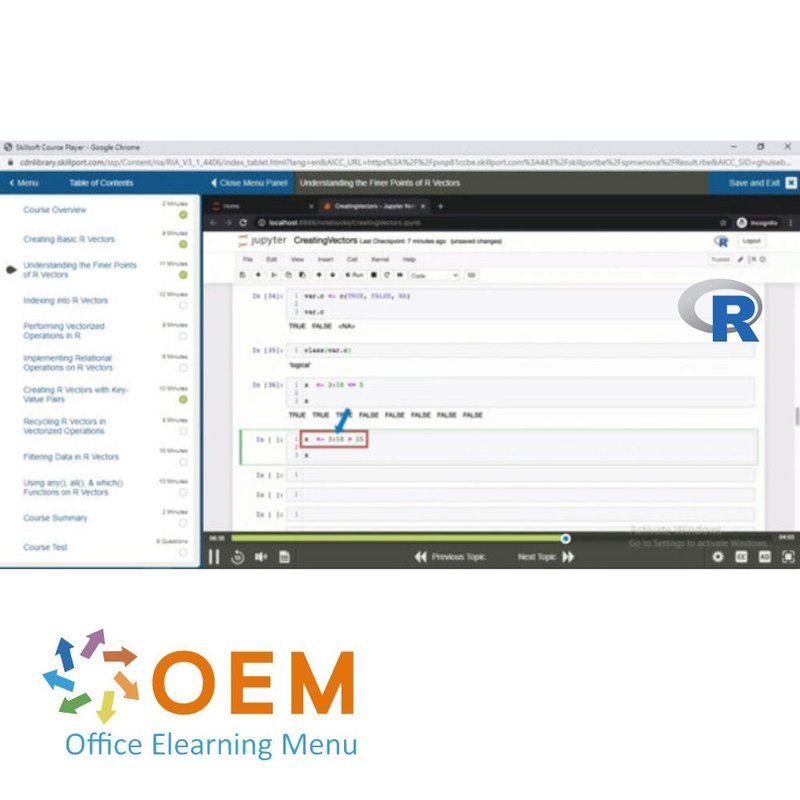
Data Analysis with R Training
Data Analysis with R E-Learning Training Certified Teachers Exam Quizzes Assessments Test Exam Live Labs Tips Tricks Certificate.
Read more- Discounts:
-
- Buy 2 for €292,04 each and save 2%
- Buy 3 for €289,06 each and save 3%
- Buy 4 for €286,08 each and save 4%
- Buy 5 for €283,10 each and save 5%
- Buy 10 for €268,20 each and save 10%
- Buy 25 for €253,30 each and save 15%
- Buy 50 for €238,40 each and save 20%
- Availability:
- In stock
- Delivery time:
- Ordered before 5 p.m.! Start today.
- Award Winning E-learning
- Lowest price guarantee
- Personalized service by our expert team
- Pay safely online or by invoice
- Order and start within 24 hours
Data Analysis with R E-Learning
R programming language is widely used for statistical analysis and modelling and data mining. In this Learning Kit, you will start by exploring the basics of R language, followed by applying the programming structures. You will then learn data analysis in R by exploring and working with Datasets in R and learn very important statistical concepts and how to apply them while analyzing and modelling your data in R.
Learning Kits are structured learning paths, mainly within the Emerging Tech area. A Learning Kit keeps
the student working toward an overall goal, helping them to achieve your career aspirations. Each part takes the student step by step through a diverse set of topic areas. Learning Kits are
made up of required tracks, which contain all of the learning resources available such as Assessments (Final Exams), Mentor, Practice Labs and of course E learning. And all resources with a 365 days access from first activation.
This Learning Kit with more than 26 hours of learning is divided into three tracks:
Course content
Module 1: Getting Started with R Programming
In this module, the focus will be on R programming for beginners. Explore the basics of R.
Courses (6 hours +):
R Programming for Beginners: Getting Started
Course: 1 Hour, 31 Minutes
- Course Overview
- Installing R on macOS
- Installing R on Windows
- Using the ? Operator in R
- Using help() and Creating Variables in R
- Using Reserved Words and Assignment Operators in R
- Using Vectors in R
- Performing Arithmetic Operations in R
- Creating Variables in R
- Using the Built-in Functions of R
- Using the Numeric Built-in Functions of R
- Recognizing the Basic Data Types in R
- Course Summary
R Programming for Beginners: Exploring R Vectors
Course: 1 Hour, 28 Minutes
- Course Overview
- Creating Basic R Vectors
- Understanding the Finer Points of R Vectors
- Indexing into R Vectors
- Performing Vectorized Operations in R
- Implementing Relational Operations on R Vectors
- Creating R Vectors with Key-Value Pairs
- Recycling R Vectors in Vectorized Operations
- Filtering Data in R Vectors
- Using any(), all(), & which() Functions on R Vectors
- Course Summary
R Programming for Beginners: Leveraging R with Matrices, Arrays, & Lists
Course: 1 Hour, 36 Minutes
- Course Overview
- Creating Matrices in R
- Naming Dimensions in R Matrices
- Performing Math Operations on R Matrices
- Implementing Matrix Multiplication in R
- Combining Matrices in R
- Performing Indexing Operations on R Matrices
- Creating Arrays in R
- Indexing into R Arrays
- Using Lists in R
- Specifying Key-Value Pairs in R Lists
- Editing Keys and Values in R Lists
- Exploring R Lists with Different Data Types
- Course Summary
R Programming for Beginners: Understanding Data Frames, Factors, & Strings
Course: 1 Hour, 53 Minutes
- Course Overview
- Creating R Data Frames
- Naming R Data Frame Dimensions & Viewing Statistics
- Indexing into R Data Frames
- Filtering Data in R Data Frames
- Combining R Data Frames
- Joining R Data Frames
- Using Factors in R to Limit Variable Values
- Creating R Data Frames with Factors
- Using Factors with tapply() and split() in R
- Viewing Counts Using Tables in R
- Working with Strings in R
- Using formatC() & sprintf() in R
- Course Summary
Assessment:
Getting Started with R Programming
Module 2: Applying and Using R Programming Structures
In this module, the focus will be on R programming structures. Explore control flow, functions, and object systems.
Courses (4 hours +)
Using R Programming Structures: Leveraging R with Control Flow & Looping
Course: 1 Hour, 13 Minutes
- Course Overview
- Conditional Branching with If Statements in R
- Using ifelse() and the Switch Statement in R
- Iterating over Data with For Loops in R
- Iterating over R Lists and Matrices with For Loops
- Using Nested For Loops in R
- Using While Loops in R
- Using Repeat Loops in R
- Performing Advanced Looping in R
- Course Summary
Using R Programming Structures: Functions & Environments
Course: 1 Hour, 41 Minutes
- Course Overview
- Creating Custom Functions in R
- Returning Data from Functions in R
- Using Named Arguments in R
- Using Default Arguments in R
- Working with First-class Functions in R
- Storing Functions & Using Them in Switch Statements
- Working with R Environments
- Creating Inner Functions in R
- Recognizing R Functions and Environments
- Working with Closures in R
- Working with Replacement Functions in R
- Course Summary
Using R Programming Structures: Object Systems
Course: 59 Minutes
- Course Overview
- Recognizing the print() Function & S3 Object System
- Identifying R Function Invocations in S
- Creating Custom Classes Using R Functions
- Extending the print() Function for R Custom Classes
- Using Reference Classes in R
- Using Member Variables and Functions in R
- Using Inheritance in Reference Classes in R
- Course Summary
Assessment:
Applying and Using R Programming Structures
Module 3: Working with Datasets In R
In this module, the focus will be on R datasets. Explore how to load, save, and transform data as well as select, filter, join, and visualize data.
Courses (6 hours +)
Datasets in R: Loading & Saving Data
Course: 1 Hour, 44 Minutes
- Course Overview
- Installing R on macOS
- Installing RStudio on macOS
- Installing R on Windows
- Installing RStudio on Windows
- Running Commands Using the RStudio Console
- Working with Panes in RStudio
- Creating a New Project and Examining Datasets
- Demonstrating and Visualizing Built-in Datasets
- Browsing Package Vignettes
- Reading from CSV Files
- Reading from Text, XML, Excel, and JSON Files
- Writing Data Out to Different File Formats
- Course Summary
Datasets in R: Transforming Data
Course: 1 Hour, 59 Minutes
- Course Overview
- Working with an In-memory SQLite Table
- Connecting to and Retrieving Results from SQLite
- Updating Results with a Persistent Database
- Dropping and Renaming Columns
- Changing Column Data Types
- Transforming Data Using the Transform Function
- Transforming Data Using the Apply Function Family
- Transforming Data Using if_else() and mutate()
- Wide Form and Long Form: Using stack() and unstack()
- Wide Form and Long Form: Using melt() and dcast()
- melt() and dcast() on a Real Dataset
- Wide Form and Long Form: Using gather() and spread()
- Course Summary
Datasets in R: Selecting, Filtering, Ordering, & Grouping Data
Course: 1 Hour, 35 Minutes
- Course Overview
- Formatting Columns to Have the Right Data Type
- Selecting Specific Rows and Columns
- Filtering Operations on Data Frame Rows
- Selecting and Filtering Using Packages in tidyverse
- Using the dplyr filter() Function
- Retrieving Samples and Top N Results
- Specifying the Correct Data Types for Columns
- Sorting Using Order and Arrange
- Grouping and Aggregations on Data Frames
- Grouping and Aggregation Using dplyr
- Course Summary
Datasets in R: Joining & Visualizing Data
Course: 47 Minutes
- Course Overview
- Joining Data Frames Using merge()
- Joining Tibbles Using Joins and Filtering Joins
- Creating Histograms and Density Curves
- Using Plots and Charts to Visualize Data
- Course Summary
Assessment:
Working with Datasets in R
Module 4: Statistical Analysis and Modeling In R
In this module, the focus will be on statistical analysis and modeling in R. Explore probability distributions, statistical tests, regression analysis, clustering, and regularized models.
Courses (9 hours +)
Statistical Analysis and Modeling in R: Working with Probability Distributions
Course: 1 Hour, 38 Minutes
- Course Overview
- Statistical Tools for Understanding Data
- Population and Sample Metric Comparisons
- Characteristics of Probability Distribution Types
- Sampling and Analyzing Uniform Distribution Data
- Sampling and Analyzing Binomial Distribution Data
- Computing Probabilities in Binomial Distributions
- Sampling and Analyzing Poisson Distribution Data
- Examining Normal and Exponential Distributions
- Interpreting QQ Plots Using R
- Using QQ Plots in R to Compare Datasets
- Course Summary
Statistical Analysis and Modeling in R: Understanding & Interpreting Statistical Tests
Course: 1 Hour, 4 Minutes
- Course Overview
- Statistical Tools for Understanding Data
- Population and Sample Metric Comparisons
- Characteristics of Probability Distribution Types
- Sampling and Analyzing Uniform Distribution Data
- Sampling and Analyzing Binomial Distribution Data
- Computing Probabilities in Binomial Distributions
- Sampling and Analyzing Poisson Distribution Data
- Examining Normal and Exponential Distributions
- Interpreting QQ Plots Using R
- Using QQ Plots in R to Compare Datasets
- Course Summary
Statistical Analysis and Modeling in R: Statistical Analysis on Your Data
Course: 2 Hours, 7 Minutes
- Course Overview
- Identifying One-sample T-test Assumptions
- Performing the One-sample T-test in R
- Performing Variations of the One-sample T-test in R
- Performing the One-sample Z-test in R
- Identifying Assumptions of the Two-sample T-test
- Running Two-sample T-tests for Equal Variances in R
- Using Welch's two-sample T-test for Unequal Variance
- Using R to Perform the Paired Samples T-test
- Checking Paired Samples T-test Assumptions Using R
- Performing the Wilcoxon Signed-rank Test Using R
- Identifying Assumptions of the ANOVA Test Using R
- Running the One-way ANOVA and Tukey HSD Tests in R
- Running the Two-way ANOVA Test for Different Models
- Parametric vs. Non-parametric Tests
- Course Summary
Statistical Analysis and Modeling in R: Performing Regression Analysis
Course: 1 Hour
- Course Overview
- The Basic Characteristics of Machine Learning Models
- Building and Evaluating Regression Models Using R
- Visualizing Data Relationships Using R
- Performing Simple Linear Regression in R
- Performing Multiple Regression in R
- Deriving Predictions Using Regression Models in R
- Building Regression Models Using Cross-validation
- Course Summary
Statistical Analysis and Modeling in R: Performing Classification
Course: 1 Hour, 37 Minutes
- Course Overview
- Recognizing and Evaluating Classification Models
- Interpreting Logistic Regression Using R
- Training and Evaluating a Logistic Regression Model
- Building a Logistic Model in R Using all Predictors
- Using R to Train a Model with Imbalanced Data
- Building and Evaluating Models with R
- Using R to Evaluate Imbalanced Data Model Types
- Using Resampling Techniques on Imbalanced Data in R
- Recognizing Decision Tree Models
- Using R to Explore and Process Data
- Visualizing Decision Trees and Performing Prediction
- Course Summary
Statistical Analysis and Modeling in R: Performing Clustering
Course: 50 Minutes
- Course Overview
- Recognizing and Evaluating Clustering Models
- Investigating and Visualizing Clustering Data in R
- Performing K-means Clustering, Interpreting Results
- Using R to Find the Optimal Number of Clusters
- Using K-means Clustering on Multi-attribute Data
- Course Summary
Statistical Analysis and Modeling in R: Building Regularized Models & Ensemble Models
Course: 1 Hour, 32 Minutes
- Course Overview
- Overfitting and Underfitting Machine Learning Models
- The Bias-Variance Trade-off
- Exploring and Understanding Data for Regression
- Performing Ordinary Least Squares (OLS) Regression
- Preparing Data for Regularized Regression Models
- Performing Ridge Regression in R
- Performing Lasso Regression in R
- Performing ElasticNet Regression in R
- Recognizing Ensemble Learning
- Using R to Explore and Visualize Data
- Performing Regression Using Decision Trees in R
- Performing Regression Using Random Forest in R
- Course Summary
Assessment:
Statistical Analysis and Modeling in R
Practice Lab: Data Science Using R
The Data Science Using R Lab will provide you with the necessary platform to gain hands on skills where you can practice different tasks related to MongoDB. You will cover areas like manipulating a data set using multiple dplyr verbs, adding the browser function to some R code to debug it, using xtable to output a table in LaTeX format, and creating an R Markdown file (.rmd) and rendering the output as html.
Language | English |
---|---|
Qualifications of the Instructor | Certified |
Course Format and Length | Teaching videos with subtitles, interactive elements and assignments and tests |
Lesson duration | 26 Hours |
Assesments | The assessment tests your knowledge and application skills of the topics in the learning pathway. It is available 365 days after activation. |
Online Virtuele labs | Receive 12 months of access to virtual labs corresponding to traditional course configuration. Active for 365 days after activation, availability varies by Training |
Online mentor | You will have 24/7 access to an online mentor for all your specific technical questions on the study topic. The online mentor is available 365 days after activation, depending on the chosen Learning Kit. |
Progress monitoring | Yes |
Access to Material | 365 days |
Technical Requirements | Computer or mobile device, Stable internet connections Web browsersuch as Chrome, Firefox, Safari or Edge. |
Support or Assistance | Helpdesk and online knowledge base 24/7 |
Certification | Certificate of participation in PDF format |
Price and costs | Course price at no extra cost |
Cancellation policy and money-back guarantee | We assess this on a case-by-case basis |
Award Winning E-learning | Yes |
Tip! | Provide a quiet learning environment, time and motivation, audio equipment such as headphones or speakers for audio, account information such as login details to access the e-learning platform. |
There are no reviews written yet about this product.
OEM Office Elearning Menu Genomineerd voor 'Beste Opleider van Nederland'
OEM Office Elearning Menu is trots genomineerd te zijn voor de titel 'Beste Opleider van Nederland' door Springest, een onderdeel van Archipel. Deze erkenning bevestigt onze kwaliteit en toewijding. Hartelijk dank aan al onze cursisten.
Reviews
There are no reviews written yet about this product.