MLOps Machine Learning Operations Training
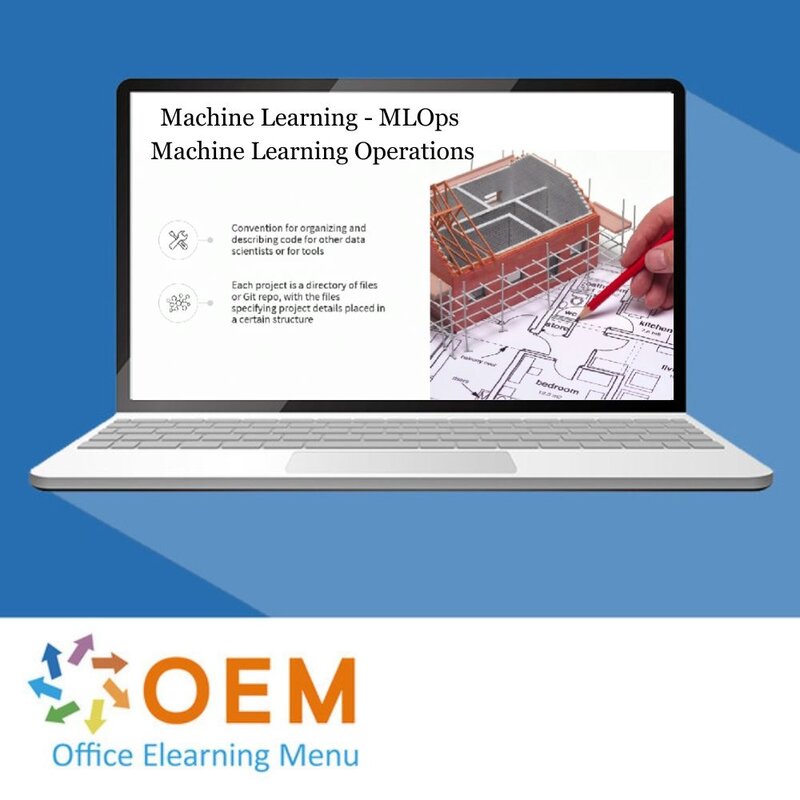
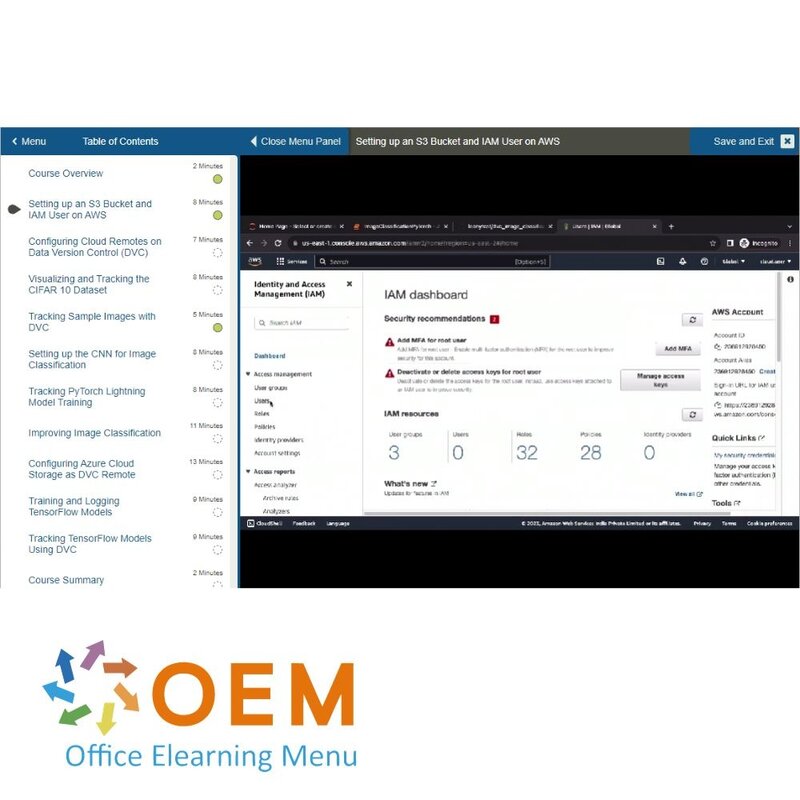
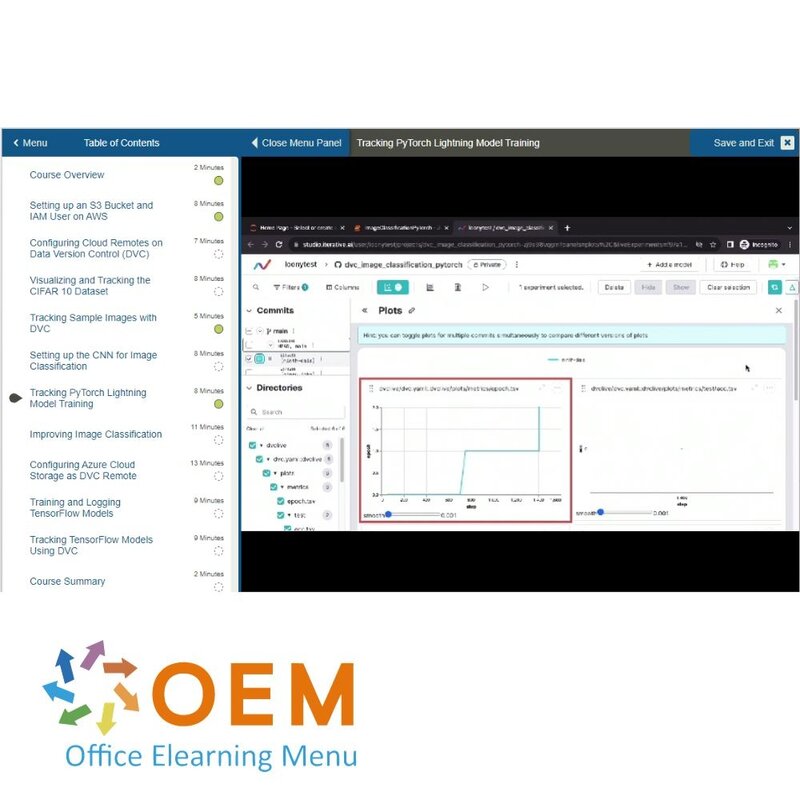
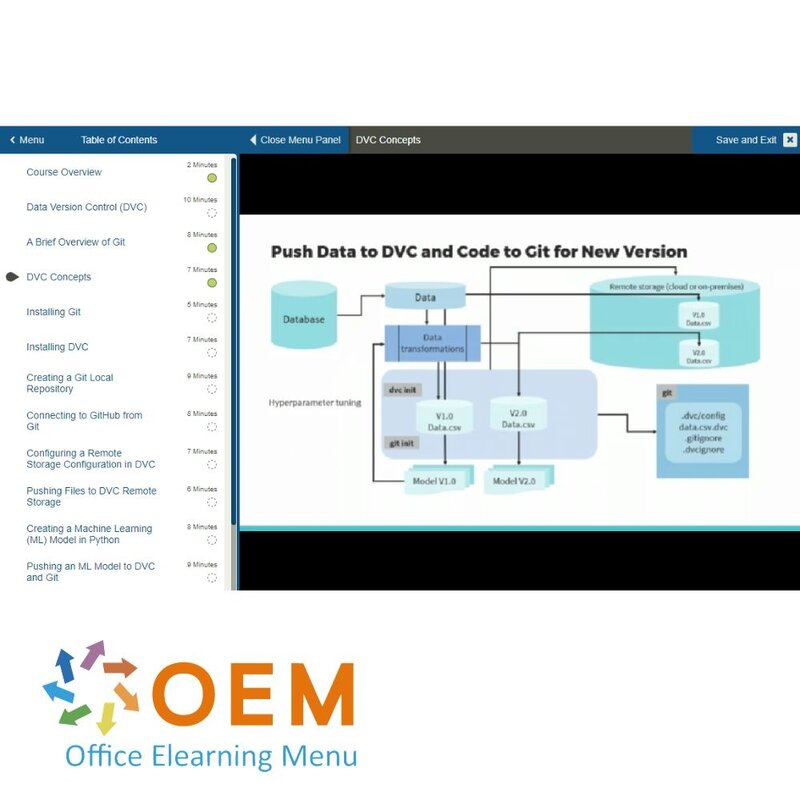
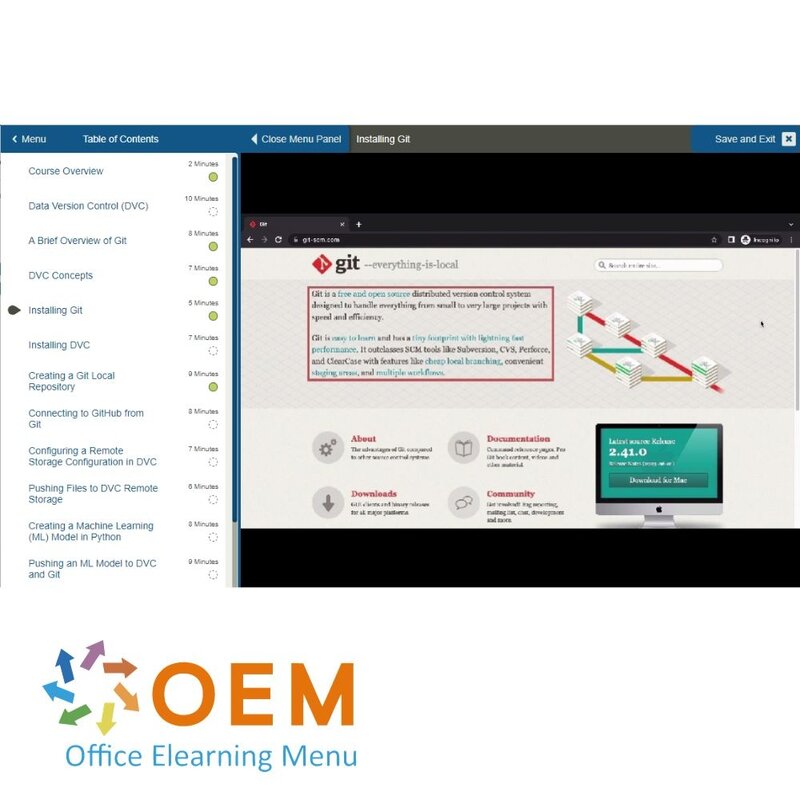
MLOps Machine Learning Operations Training
MLOps Machine Learning Operations E-Learning Training Certified teachers Quizzes Assessments Tips tricks and Certificate.
Read more- Discounts:
-
- Buy 2 for €194,04 each and save 2%
- Buy 3 for €192,06 each and save 3%
- Buy 4 for €190,08 each and save 4%
- Buy 5 for €188,10 each and save 5%
- Buy 10 for €178,20 each and save 10%
- Buy 25 for €168,30 each and save 15%
- Buy 50 for €158,40 each and save 20%
- Availability:
- In stock
- Delivery time:
- Ordered before 5 p.m.! Start today.
- Award Winning E-learning
- Lowest price guarantee
- Personalized service by our expert team
- Pay safely online or by invoice
- Order and start within 24 hours
MLOps Machine Learning Operations E-Learning
Bridge the gap between data science and deployment with MLOps.
Embark on a transformative journey into the world of MLOps (Machine Learning Operations)—the intersection of data science, DevOps, and engineering. This training equips you with the knowledge and skills needed to take machine learning models from experimental notebooks to production-grade pipelines.
In this course, you’ll explore:
- The fundamentals of MLOps and how it differs from standard ML workflows
- Tools and frameworks for version control, CI/CD, deployment, and monitoring
- Building scalable, reproducible, and automated ML pipelines
- Best practices in model governance, data security, and infrastructure
- Fostering collaboration between data science, engineering, and operations
Built on an Agile learning framework, this course promotes continuous improvement through practical, iterative learning.
Why Choose This Training?
- Combines ML, DevOps, and IT operations in a unified training path
- Hands-on practice with tools for automation, deployment, and model monitoring
- Learn to scale and operationalize machine learning effectively
- Covers the full ML lifecycle from experimentation to post-deployment
- Includes e-learning, mentor support, labs, assessments & 365-day access
Who Should Enroll?
This course is perfect for:
- Data scientists aiming to bring their models into production
- ML and DevOps engineers looking to master MLOps tools and practices
- IT professionals and AI students seeking hands-on MLOps experience
- Teams and organizations ready to scale ML operations across departments
This Learning Kit with more than 23 hours of learning is divided into three tracks:
Course content
Track 1: Intro to MLOps
In this track of the MLOps Aspire Journey, the focus will be on understanding the fundamental concepts and principles that underpin this transformative field. Explore the evolution of MLOps, dissect the MLOps workflow, and delve into the challenges and best practices that await you on this exciting journey.
Courses (1½ hour):
Getting Started with MLOps
Course: 1 Hour, 27 Minutes
- Course Overview
- Introducing MLOps
- What's Different About MLOps?
- Factors Affecting Machine Learning (ML) Models in Production
- Solving Machine Learning Problems
- The Machine Learning Canvas
- End-to-end Machine Learning Workflow
- ML Workflow Architectural Patterns
- Stages in MLOps Maturity Level
- Stages in MLOps Maturity Level 1 and 2
- Course Summary
Track 2: MLFlow
In this track of the MLOps Aspire Journey, the focus will be on how to track, manage, and deploy your machine learning models efficiently. From MLFlow tracking and models to model deployment and CI/CD integration, this track empowers you with essential MLOps skills.
Courses (11 hours +)
MLOps with MLflow: Getting Started
Course: 1 Hour, 27 Minutes
- Course Overview
- Introducing MLflow
- The Machine Learning Workflow
- Understanding Model Deployment
- MLflow Concepts and Components
- The Features of MLflow
- Model Signature
- MLflow Tracking
- Installing MLflow
- Installing MLflow in a Virtual Environment
- Viewing the MLflow User Interface (UI) and Directory Structure
- Setting up an MLflow Virtual Environment for Jupyter
- Course Summary
MLOps with MLflow: Creating & Tracking ML Models
Course: 1 Hour, 45 Minutes
- Course Overview
- Loading, Cleaning, and Visualizing Data for Machine Learning
- Viewing Data Statistics with Pandas Profiling
- Creating an MLflow Experiment
- Creating an MLflow Run and Logging Artifacts
- Creating a Run in a With Block and Viewing Run Info
- Creating Multiple Runs for Different Models
- Running Polynomial and Random Forest Regression Models
- Comparing and Visualizing Models
- Using MLflow Autologging
- Viewing Autologged Metrics and Artifacts
- Exploring the conda.yaml File
- Configuring Autologging to Log Test Metrics
- Comparing MLflow Models Using the UI
- Course Summary
MLOps with MLflow: Registering & Deploying ML Models
Course: 1 Hour, 57 Minutes
- Course Overview
- Visualizing and Cleaning Data
- Creating an Experiment from the MLflow U
- Running a Classification Model and Viewing its Metrics
- Analyzing Model Insights Using SHAP
- Running Multiple Classification Models
- Comparing Models Programmatically
- Registering an MLflow Model
- Modifying Registered Model Versions
- Registering Another Model and Viewing the Registered Model
- Serving Models to a Local REST Endpoint
- Creating an Azure Machine Learning (Azure ML) Account
- Registering a Model on Azure
- Accessing Models through Azure REST Endpoints
- Course Summary
MLOps with MLflow: Hyperparameter Tuning ML Models
Course: 1 Hour, 37 Minutes
- Course Overview
- Understanding How MLflow Works with Databricks
- Creating a Databricks Workspace and Cluster
- Uploading a File to DBFS and Running a Model from Databricks
- Setting Up the Objective Function for Hyperparameter Tuning
- Understanding the Objective Function and Viewing the Runs
- Defining the Search Space and Search Algorithm
- Running a Hyperparameter Tuning Model and Viewing the Results
- Setting Up SQLite and Using MLflow with SQLite
- Performing Data Cleaning and Building a Regression Model
- Building and Tracking a Regression Model Using statsmodels
- Course Summary
MLOps with MLflow: Creating Time-series Models & Evaluating Models
Course: 1 Hour, 23 Minutes
- Course Overview
- Cleaning Data for a Time-series Model
- Training a Model and Viewing the Artifacts
- Performing Cross-validation and Evaluating Performance
- Cleaning Data and Performing Encoding
- Creating a Machine Learning Model and Setting Up Model Evaluation
- Evaluating a Model and Analyzing the Lift Curve
- Understanding the Precision-Recall Curve and Beeswarm Charts
- Using a Metric Threshold to Evaluate a Model
- Course Summary
MLOps with MLflow: Tracking Deep Learning Models
Course: 1 Hour, 31 Minutes
- Course Overview
- Preprocessing Image Data for Machine Learning and Viewing the Images
- Training and Running an Image Classification Model
- Viewing Performance and Registering an Image Classification Model
- Deploying a Model to Azure, Viewing It, and Making Predictions
- Exploring PyTorch and Viewing Images for Machine Learning
- Setting Up and Running an Image Classification Model
- Viewing Model Performance, Serving It, and Making Predictions
- Running a Sentiment Analysis Model and Viewing Logged Artifacts
- Course Summary
MLOps with MLflow: Using MLflow Projects & Recipes
Course: 2 Hours, 8 Minutes
- Course Overview
- MLflow Projects
- Creating, Viewing, and Modifying an MLflow Project
- Creating and Running an Experiment for a Project and Viewing Results
- MLflow Recipes
- Creating an MLflow Recipe and Exploring Its Files
- Using the MLflow Regression Template
- Viewing and Modifying Files in a Recipe
- Modifying the train.py and the custom_metrics.py File
- Working with the recipe.yaml and local.yaml Files
- Creating a Recipe and Viewing the Recipe Pipeline
- Running Our Recipe and Viewing Model Evaluation Results
- Validating Models Based on a Metrics Threshold
- Setting up a Classification Recipe and Modifying the YAML Files
- Running a Classification Recipe and Viewing the Results
- Training Models with Data from DBFS and Delta Lakes
- Course Summary
Track 3: Data Version Control
In this track of the MLOps Aspire Journey, you will discover the power of Data Version Control (DVC) and its role in simplifying experiment tracking, model management, and automation in MLOps. Explore DVC's VS Code extension, command-line tools, and open-source version control system. Learn to streamline your machine learning workflows and enable continuous machine learning with DVC.
Courses (9 hours +)
MLOps with Data Version Control: Getting Started
Course: 1 Hour, 52 Minutes
- Course Overview
- Data Version Control (DVC)
- A Brief Overview of Git
- DVC Concepts
- Installing Git
- Installing DVC
- Creating a Git Local Repository
- Connecting to GitHub from Git
- Configuring a Remote Storage Configuration in DVC
- Pushing Files to DVC Remote Storage
- Creating a Machine Learning (ML) Model in Python
- Pushing an ML Model to DVC and Git
- Viewing the Files Committed to GitHub
- Running and Pushing a Different Model Version
- Reverting to Previous Code Versions in Git
- Course Summary
MLOps with Data Version Control: Working with Pipelines & DVCLive
Course: 2 Hours, 12 Minutes
- Course Overview
- Setting up a Machine Learning (ML) Pipeline Stage
- Adding a Stage to a Data Version Control (DVC) Pipeline
- Using the dvc.lock File
- Executing a DVC Pipeline
- Setting up a DVC Project for Regression Analysis
- Setting up Iterative Studio and DVCLive
- Setting up Data for Visualizing and Tracking Using DVC
- Logging Plots Using DVCLive
- Logging and Tracking Images Using DVCLive
- Tracking Experiments with DVCLive
- Pushing Experiment Files to DVC
- Committing a Pull Request to Merge Experiment Details
- Running and Tracking a kNN Regression Experiment with DVC
- Tracking Model Artifacts
- Registering Models with the Studio Registry
- Course Summary
MLOps with Data Version Control: Tracking & Serving Models with DVC & MLEM
Course: 1 Hour, 54 Minutes
- Course Overview
- Preprocessing Data for Churn Prediction
- Tracking and Comparing Logistic Regression Experiments
- Tracking and Comparing Random Forest Experiments
- Tracking an XGBoost Experiment
- Tracking Artifacts and Registering a Classification Model
- Setting up the DVC Project for Hyperparameter Tuning
- Performing Hyperparameter Tuning Using Optuna
- MLEM
- Extracting Model Codification Using MLEM
- Using MLEM to Serve Models Locally on FastAPI
- Installing and Setting up Docker
- Deploying a Model in a Docker Container
- Getting Predictions from a Docker Hosted Model
- Course Summary
MLOps with Data Version Control: Tracking & Logging Deep Learning Models
Course: 1 Hour, 31 Minutes
- Course Overview
- Setting up an S3 Bucket and IAM User on AWS
- Configuring Cloud Remotes on Data Version Control (DVC)
- Visualizing and Tracking the CIFAR 10 Dataset
- Tracking Sample Images with DVC
- Setting up the CNN for Image Classification
- Tracking PyTorch Lightning Model Training
- Improving Image Classification
- Configuring Azure Cloud Storage as DVC Remote
- Training and Logging TensorFlow Models
- Tracking TensorFlow Models Using DVC
- Course Summary
MLOps with Data Version Control: Creating & Using DVC Pipelines
Course: 1 Hour, 21 Minutes
- Course Overview
- Configuring a DVC Project for an ML Pipeline
- Tracking Training Data with DVC
- Adding the Data Process Stage to the ML Pipeline
- Executing Pipeline Stages
- Adding a Train Stage to the ML Pipeline
- Executing the Data Process and Train Stages
- Adding and Executing the Evaluate Stage in a Pipeline
- Eliminating a Duplicate dvc.yaml File
- Running DVC Experiment Pipelines
- Queueing and Running Experiments
- Course Summary
MLOps with Data Version Control: CI/CD Using Continuous Machine Learning
Course: 1 Hour, 3 Minutes
- Course Overview
- Continuous Machine Learning (CML)
- Configuring Google Drive as DVC Remote Storage
- Authorizing DVC to Use Google Drive
- Creating DVC Pipeline
- Configuring a CML Workflow for CI/CD
- Triggering CI/CD with Git Push
- Viewing Metric and Plot Comparisons with CML Reports
- Course Summary
Assessment:
- Final Exam: MLOps
Language | English |
---|---|
Qualifications of the Instructor | Certified |
Course Format and Length | Teaching videos with subtitles, interactive elements and assignments and tests |
Lesson duration | 23:08 Hours |
Assesments | The assessment tests your knowledge and application skills of the topics in the learning pathway. It is available 365 days after activation. |
Online Virtuele labs | Receive 12 months of access to virtual labs corresponding to traditional course configuration. Active for 365 days after activation, availability varies by Training |
Online mentor | You will have 24/7 access to an online mentor for all your specific technical questions on the study topic. The online mentor is available 365 days after activation, depending on the chosen Learning Kit. |
Progress monitoring | Yes |
Access to Material | 365 days |
Technical Requirements | Computer or mobile device, Stable internet connections Web browsersuch as Chrome, Firefox, Safari or Edge. |
Support or Assistance | Helpdesk and online knowledge base 24/7 |
Certification | Certificate of participation in PDF format |
Price and costs | Course price at no extra cost |
Cancellation policy and money-back guarantee | We assess this on a case-by-case basis |
Award Winning E-learning | Yes |
Tip! | Provide a quiet learning environment, time and motivation, audio equipment such as headphones or speakers for audio, account information such as login details to access the e-learning platform. |
There are no reviews written yet about this product.
OEM Office Elearning Menu Top 2 in ICT-trainingen 2024!
OEM Office Elearning Menu is trots op de tweede plaats in de categorie ICT-trainingen 2024 bij Beste Opleider van Nederland (Springest/Archipel). Dank aan al onze cursisten voor hun vertrouwen!
Reviews
There are no reviews written yet about this product.